3D Magnetic Resonance Fingerprinting (MRF) with Hybrid Sliding-Window and GRAPPA Reconstruction
We are pleased to announce the release of our 3D MRF sequence and off-line reconstruction packages for fast quantitative imaging applications. This package is developed by research groups at Stanford University, Martinos Center for Biomedical Imaging / MGH, and Center for Brain Imaging Science and Technology (CBIST) at Zhejiang University (ZJU).
Pulse sequence for 3D MRF is only available for the Siemens Prisma and Skyra Scanners with VE11C/E platforms. The off-line reconstruction script is based on MATLAB 2014b or later versions.
To request the software, instructions and example protocols, please navigate to martinos.org/c2p
Introduction:
Magnetic Resonance Fingerprinting (MRF) (1) has shown great potential for efficient multi-parameter mapping. 3D-MRF acquisitions (2–6) enjoy an SNR efficiency benefit over their 2D counterparts, and could help achieve high SNR at high resolutions. However, high resolution imaging with whole-brain coverage can lead to lengthy scans which, in turn, increases motion sensitivity. To accelerate 3D-MRF, our work (6) combines stack-of-spiral acquisition with hybrid sliding-window (7) and GRAPPA (8) (SW+GRAPPA) reconstruction (Fig.1). This enables >10x acceleration through
- 3-fold acceleration in kz encoding and
- 3.6-fold reduction in the number of TRs for pattern matching.
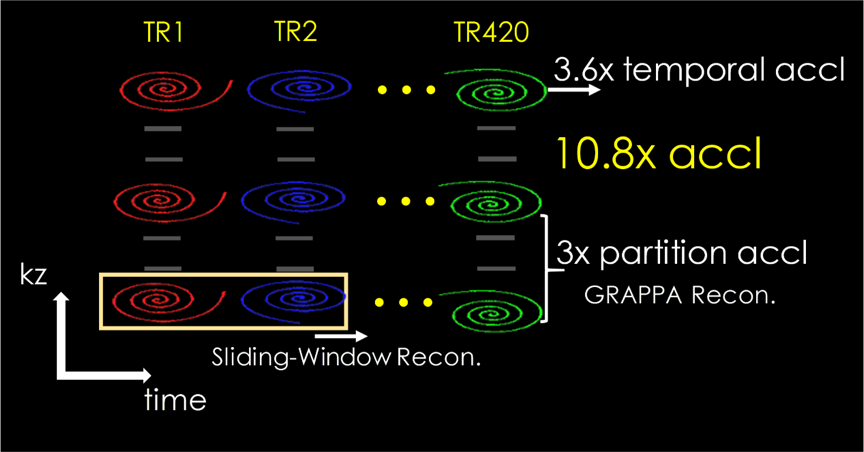
Sequence:
3D FISP sequence (9) was implemented for MRF. The partition-by-partition sampled sequence that also incorporates a low-flip-angle training data acquisition into the wait period. The total acquisition time for each partition is 8 seconds for a 420 time-points (tps) acquisition.
SW+GRAPPA recon:
3D coil sensitivity profiles were estimated from the center fully-sampled k-space region of the training data using ESPIRiT (10-12). SW and NUFFT (13-15) were used to remove in-plane aliasing and create a Cartesian dataset that is fully sampled in-plane. This then allows for a direct application of Cartesian GRAPPA reconstruction to overcome Rz acceleration. More details can be found in (6).
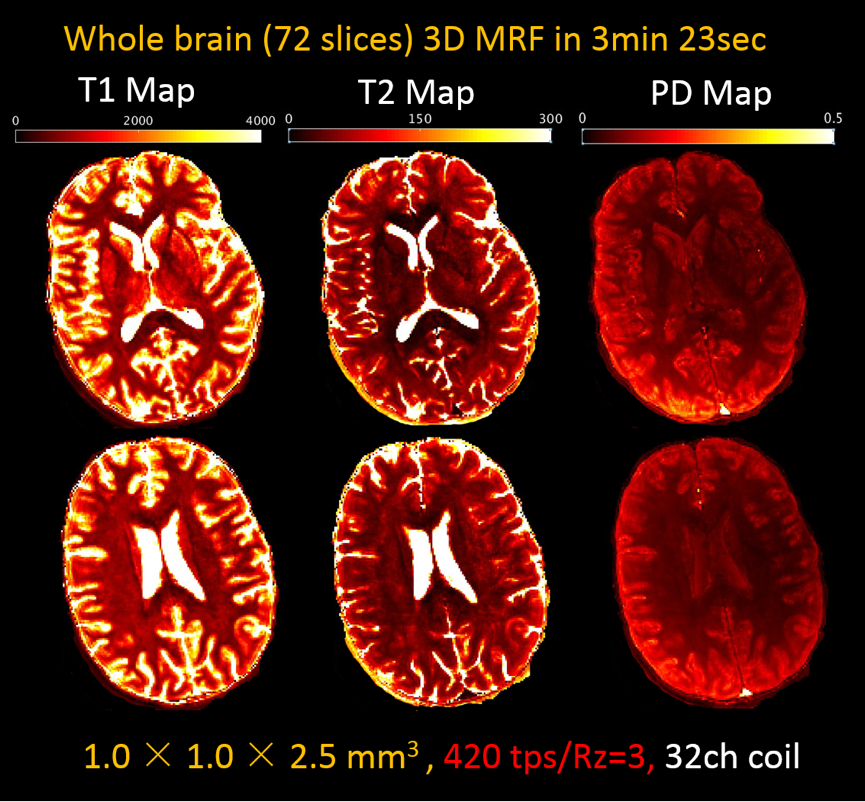
Dictionary generation and pattern recognition:
The dictionary was generated by 2-step extended phase graph (EPG) (16) simulation using variable TRs and FAs. The effect of the low-flip-angle GRAPPA training acquisitions and the T1 recovery during the waiting period between each partition were also included in the dictionary generation process. The SW+GRAPPA reconstructed 3D volumes were then normalized and pattern matched voxel-wise to the corresponding dictionary using the maximum inner product method to obtain T1 and T2 maps.
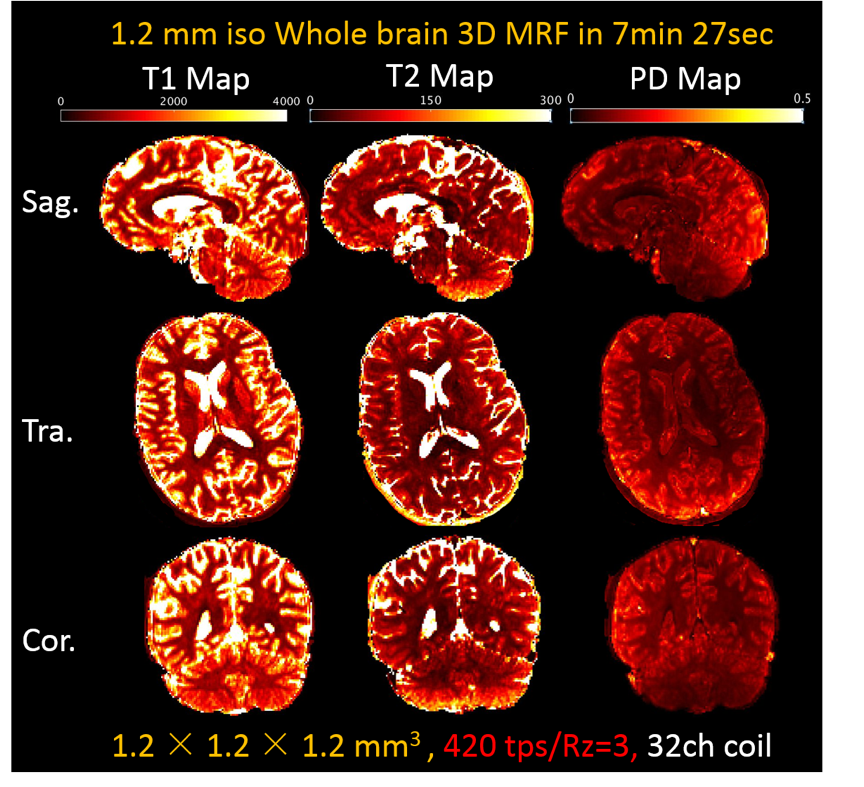
References:
- Ma D, Gulani V, Seiberlich N, Liu K, Sunshine JL, Duerk JL, Griswold MA. Magnetic resonance fingerprinting. Nature 2013;495:187–192.
- Ma D, Hamilton J, Jiang Y, Seiberlich N, Griswold MA. Fast 3D Magnetic Resonance Fingerprinting (MRF) for Whole Brain Coverage in Less Than 3 Minutes. In: Proceedings of the 24th Annual Meeting of ISMRM, Singapore. Singapore; 2016. p. 3180.
- Ma D, Pierre EY, Jiang Y, Schluchter MD, Setsompop K, Gulani V, Griswold MA. Music-based magnetic resonance fingerprinting to improve patient comfort during MRI examinations. Magn. Reson. Med. 2016;75:2303–2314. doi: 10.1002/mrm.25818.
- Buonincontri G, Sawiak SJ. MR fingerprinting with simultaneous B1 estimation. Magn. Reson. Med. 2016;76:1127–1135. doi: 10.1002/mrm.26009.
- Ma D, Jiang Y, Chen Y, McGivney D, Mehta B, Gulani V, Griswold M. Fast 3D magnetic resonance fingerprinting for a whole-brain coverage. Magn. Reson. Med. 2017. doi: 10.1002/mrm.26886.
- Liao C, Bilgic B, Manhard MK, Zhao B, Cao X, Zhong J, Wald LL, Setsompop K. 3D MR fingerprinting with accelerated stack-of-spirals and hybrid sliding-window and GRAPPA reconstruction. 2017. doi: 10.1016/j.neuroimage.2017.08.030.
- Cao X, Liao C, Wang Z, Chen Y, Ye H, He H, Zhong J. Robust sliding-window reconstruction for Accelerating the acquisition of MR fingerprinting. Magn. Reson. Med. 2016. doi: 10.1002/mrm.26521.
- Griswold MA, Jakob PM, Heidemann RM, Nittka M, Jellus V, Wang J, Kiefer B, Haase A. Generalized autocalibrating partially parallel acquisitions (GRAPPA). Magn. Reson. Med. 2002;47:1202–1210. doi: 10.1002/mrm.10171.
- Jiang Y, Ma D, Seiberlich N, Gulani V, Griswold MA. MR fingerprinting using fast imaging with steady state precession (FISP) with spiral readout. Magn. Reson. Med. 2015;74:1621–1631. doi: 10.1002/mrm.25559.
- Uecker M, Lai P, Murphy MJ, Virtue P, Elad M, Pauly JM, Vasanawala SS, Lustig M. ESPIRiT - An eigenvalue approach to autocalibrating parallel MRI: Where SENSE meets GRAPPA. Magn. Reson. Med. 2014;71:990–1001. doi: 10.1002/mrm.24751.
- BART toolbox v0.2.06 doi: 10.5281/zenodo.13931
- Uecker M, Ong F, Tamir JI, Bahri D, Virtue P, Cheng JY, Zhang T, Lustig M. Berkeley advanced reconstruction toolbox. In: Proc. Intl. Soc. Mag. Reson. Med. Vol. 23. ; 2015. p. 2486.
- Michigan Image Reconstruction Toolbox (MIRT)
- Fessler JA. On NUFFT-based gridding for non-Cartesian MRI. J. Magn. Reson. 2007;188:191–195.
- Fessler JA and Sutton BP. Nonuniform fast Fourier transforms using min-max interpolation. IEEE Transactions on Signal Processing. 2003; 51(2). 560-574
- Weigel M. Extended phase graphs: Dephasing, RF pulses, and echoes‐pure and simple. J. Magn. Reson. Imaging 2015;41:266–295.